February 16, 2024
Radiologists balance caution and optimism about AI
by Digital Health Insights 7 minutes
AI is moving in on image-heavy specialties like radiology, but clinicians don’t have to worry about being rendered obsolete any time soon.
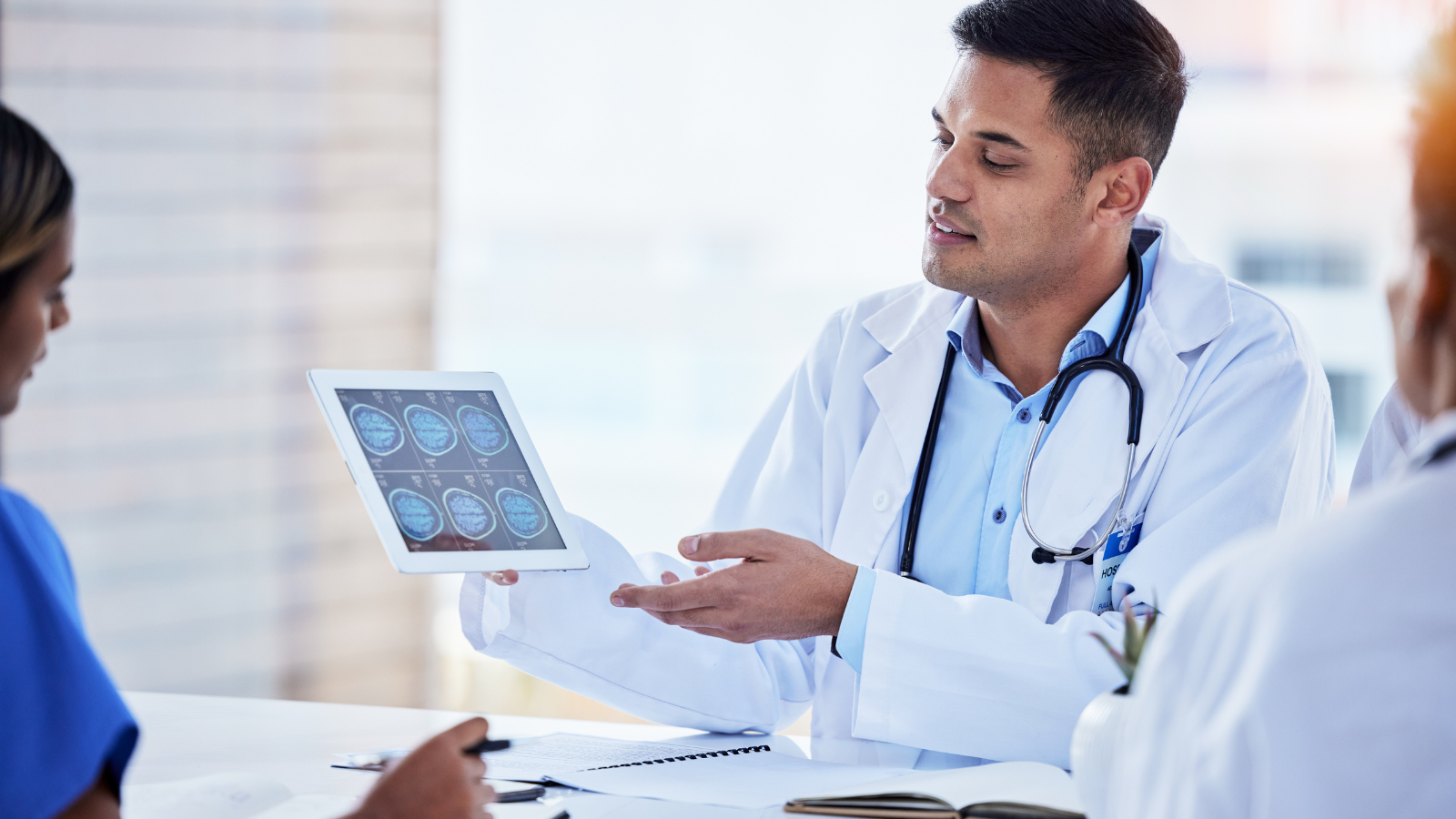
In 2016, AI expert Geoffrey Hinton famously stated that there was no further need to train radiologists in a specialty that was going to be completely taken over by artificial intelligence within the next decade.
While he has since softened his statements and narrowed his predictions to the interpretation of medical scans, the commentary has mirrored the tone for discussions about the role of AI in image-heavy specialties for nearly a decade – so much so that nearly a quarter of prospective radiologists are reconsidering their career path and 42% of medical students believe AI poses a significant threat to the radiology job market.
They may not be wrong to be concerned that AI is going to change what it means to be a radiologist. AI is definitely coming to the specialty, and quickly. Of the approximately 700 AI-enabled medical devices and algorithms cleared by the FDA as of late 2023, more than 70% are targeted to radiology use cases, far exceeding the number aimed at pathology, ophthalmology, or cardiovascular care.
But despite some algorithms approaching or even exceeding the performance of humans on specific image reading tasks, radiologists aren’t packing their bags just yet. That’s partially because there’s a lot more to radiology than looking at pictures, but also because most radiologists reject the all-or-nothing premise of the issue.
AI isn’t intended to replace them. It’s designed to make them better at what they do – and for those who embrace the bright side of what AI has to offer, the process is already well underway.
“Computer aided detection (CAD) is nothing new for our specialty,” said Dr. Tim O’Connell, a practicing radiologist in Vancouver, Canada, and CEO of Emtelligent, an AI company focused on medical documentation improvement, in an interview with Digital Health Insights. “It’s been around for 20 years or so, in various forms, particularly for breast cancer. I, for one, am really looking forward to having great AI models sit over my shoulder for even more use cases to help make sure that I am identifying the right dots in a sea of dots on an image.”
It’s going to make me more confident as a diagnostician, and it’s going to make the health system more efficient. Anything we can do to safely improve care delivery is going to be a good thing for the health system and for patients, so why wouldn’t I want to use it?
Getting realistic about the intersection of AI and radiology
“When Geoffrey Hinton amended his predictions, he acknowledged that radiologists do a lot more than sit in dark rooms and look at pictures all day,” O’Connell noted. “Both interventional radiologists and diagnostic radiologists do a large number of procedures on patients, as well, such as image-guided biopsies and complex vascular procedures.”
“So even if AI were to come in tomorrow and take over all of the work that happens in those dark rooms, there will always be an absolutely crucial role for radiologists in patient care.”
Nevertheless, there are likely to be some big changes in store for the discipline over the next five to ten years – if algorithm developers can solve some fundamental problems with the complexity of analyzing highly detailed images from a wide array of testing modalities.
“There may be an opportunity for fully replacing humans in some simple diagnostic tasks, such as looking at wrist x-rays,” said O’Connell. “But for a CT scan that might include hundreds or thousands of images, there are a lot of different challenges there.”
“For example, some CT scanners will take images with 2 millimeter thick slices, while others might do 5 millimeters. The resolution and detail on each of those is going to be different. Even using one manufacturer’s imaging equipment versus another is going to produce images of different quality and character that could affect the interpretation of results. Training an AI model to account for all of those factors is an enormous challenge, and it could produce very significant differences in accuracy and reliability depending on the composition of the dataset used for training.”
As new algorithms are rolled out – including homegrown tools that are increasingly popular among radiologists, according to a 2021 survey from the American College of Radiologists (ACR) – clinicians and healthcare leaders are going to need to monitor these potential issues with the reliability and accuracy of suggestions or reports produced by AI models.
Most radiologists agree that oversight must be a fundamental part of the adoption process, ACR members agree, with approximately 60% of respondents indicating they would want external validation of models across representative datasets. The same number added they would prefer to be able to assess the performance of an AI model on their own patient data before deploying it in real-world situations.
“Process management, training, and governance are going to be key for success, especially since we’re starting to see radiologists using a whole toolbox of different algorithms for different use cases, each with their own strengths and weaknesses,” agreed O’Connell. “You might have one for chest x-rays, one for mammography, and one for head CTs, and if you don’t have the training or experience to use each of them appropriately, that could produce a lot of problems.”
“Maintaining the work environment for these tools, getting radiologists involved in validation and training, and ensuring clear communication with the people who are using these tools will be vital for making sure we can make the most of what AI has to offer us.”
A complementary future for AI and human radiologists
O’Connell, along with many of his colleagues in the United States and elsewhere, are optimistic about the opportunity to augment their skillset with AI.
Approximately a quarter of respondents to the ACR survey in 2021 were planning to bring new AI tools onboard within the next one to five years, adding to the 30% of practices that had already integrated advanced models into their workflows.
The ACR’s Data Science Institute has even launched an AI lab to help clinicians get involved in creating, testing, and tailoring models for creative, high-value use cases.
“There are so many exciting areas where AI can assist radiologists, and not just in reading static images,” O’Connell said. “For example, when doing a procedure like an ultrasound-guided biopsy, the tip of the needle can be very difficult to see.”
“Obviously, we need to know exactly where that needle is going, because that’s what can potentially cause injury. There are some folks working on using AI to enhance the visibility of the needle tip on the ultrasound image so we can always see what’s happening clearly. My response is, ‘yes please, sign me up!’ Anything that can reduce my stress, reduce the chance of injury to the patient, and make it easier to get the result we want is something I want to use.”
Integrating AI into radiology will require a measured approach that combines careful training and monitoring with a willingness to embrace a different definition of what it means to be a diagnostician and practitioner in a digitally driven world.
To make the most of what AI has to offer in the immediate future while preparing for long-term changes, “radiologists should do what they’ve always traditionally been very good at doing, which is cautiously implementing new technologies hand-in-hand with industry and regulators,” O’Connell concluded. “We need to keep an open mind while still being diligent about safety, efficacy, and validation.”
“There is a bright and wonderful future for AI, as long as executives, developers, and clinicians can work together to adopt the right tools for the right reasons. If clinicians push back and say they don’t think something is safe, or it is going to hurt their workflow, we need to listen to those concerns and make adjustments accordingly so we can use AI to its full potential.”